Should you believe innocent looks or statistics? I hope you side with statistics after reading this blog. Otherwise it would mean I have failed as an educator. Well, one day I came home and found my dogs near a bunch of chewed books. To be fair, I didn’t catch them red-handed, but it was “obvious” that they were the ones who chewed the books. Evidence is out there. Apart from innocent looks, they sounded smart and the picture below says it all, doesn’t it?
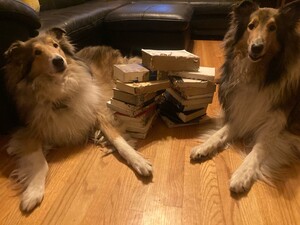
This situation reminded me of my statistics course where I taught concepts like hypothesis testing, statistical significance and p-value. In hypothesis testing, we reach a statistical significance when we get a small p-value relative to a pre-determined alpha value. The standard for confirming statistical significance is a p-value smaller than 0.05, although smaller or larger p-values are also used.
The small p-value means that the null hypothesis is unlikely given the observed data. To be clear, the p-value does not tell us about the probability that the hypothesis is true or false. Rather, it tells us how unusual the data is assuming the null hypothesis is true. Therefore, rejecting the null hypothesis doesn’t necessarily mean that the alternative hypothesis is correct. It only suggests that we have sufficient evidence to reject the null hypothesis.
Now, let’s break this down in the case of dogs chewing books. My mom loves the dogs, and she argues that dogs are innocent. We might view this as a null hypothesis. But what about catching them near a pile of the chewed books? Let’s accept this as an alternative hypothesis. Then, we will get the following scenario:
H0: My dogs don’t chew books. 🡺 Null hypothesis
H1: My dogs chew books. 🡺Alternative hypothesis
In our scenario, the p-value is the probability of finding dogs standing by the chewed books under the assumption that the dogs don’t chew books (null hypothesis). What is the chance of finding dogs near the chewed books if the assumption that my dogs don’t chew books is true? Very small, right? (Well unless my door was open, and the neighbor’s dogs had access to my house; this is Type I error. Or if we believe in conspiracy theories that the Martians secretly came and did that while I wasn’t at home).
Indeed, we should conclude that the p-value is very small here since it is less likely to find dogs standing by the chewed books if the dogs don’t really chew books. Think of finding dogs near chewed books as data. We test our alternative hypothesis against this data. We would more likely reject the null hypothesis and confirm the alternative hypothesis that the dogs actually chew the books.
Conceptually, the p-value is the probability or proportion of obtaining test results at least as extreme as the result actually observed, assuming that the null hypothesis is true. In this analogy, we made our decision qualitatively. Statistical tests provide a number, which is compared against a previously determined alpha value. For example, if we set the alpha value to 0.05 and then get a p-value smaller than the alpha value, then we reject the null hypothesis and conclude that the results are significant.
P-value approach stands at the core of statistical significance testing. It is widely used in academic research. Whether it is elementary statistics tests such as t-tests, chi-square tests, and ANOVA or advanced regression analysis and many others, almost all statistical tests provide us with p-value, which tells us how much our research findings are just by chance. If we find this randomness very low, we should then have confidence that the results are because of hypothesized independent variables. However, you might find p-value less useful in terms of predicting outcomes, a situation that some call “perils of policy by p-value”. It is for this reason that private sector relies less on p-value approach as investors care more about prediction. But this is the topic of another blog.
Namig Abassov, Digital Humanities Data Analyst
Questions about Data Science and Analytics? Reach out to us at datascience@asu.edu